“There is no cost to getting things wrong. The cost is not getting them published.”
– Brian Nosek
In the 1990s, a small biotechnology company tried to develop food crops with enhanced traits. Taller shoots, fatter fruits, drought resistance — that sort of thing. The company designed experiments and ran field trials for 15 years.
Results were published in peer-reviewed journals. Everything seemed on track. The small company convinced a large, multinational seed company to license the improved strains — and that’s when the problems started.
Faced with inconsistent results, the small company hired a statistician to review the data. The verdict: It was impossible to tell whether the engineered plants were statistically superior to wildtype. The company took this as a null result and said "At least now we know it doesn’t work!" (Perhaps they were mindful of the famous story about Thomas Edison failing in his first 10,000 attempts at inventing the lightbulb.) The statistician replied: “Actually, the data you gathered don't even let you conclude that.”
Years of effort and $70 million, down the drain. After testing all those lightbulbs, they had forgotten to plug them in.
This same story has played out many times in drug development, too. Most major randomized, controlled trials before the year 2000 showed therapeutic benefits (Figure 1), according to data on NHLBI-funded trials for cardiovascular disease between 1970 and 2012. But after the year 2000, when the agency codified hypothesis pre-registration, trials mostly ended in null results. Useless drugs, with possible side effects, had almost certainly been approved, manufactured, and swallowed by Americans.
Scientists are taught pre-recorded hypotheses and rigorous statistics — those fundamental tools of good research — but apply them inconsistently. In this sense, they can learn from the law.
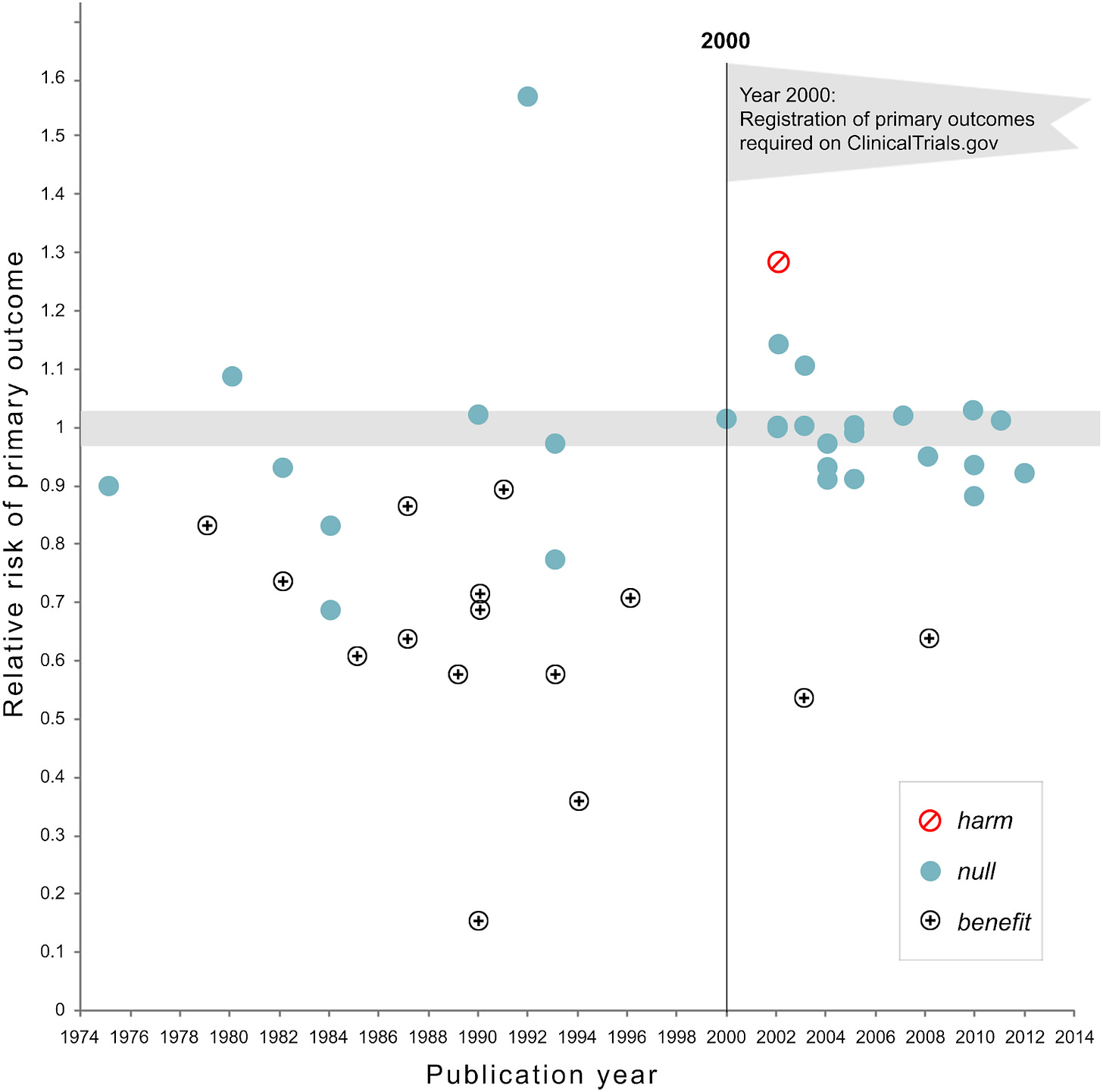
The vast majority of neuroimaging studies are underpowered and rarely produce results above noise. The odds that an average neuroscience study is true is 50-50 or lower, according to a 2013 review. And an estimated 50% of studies in biomedicine “have statistical power in the 0–10% or 11–20% range, well below the minimum of 80% that is often considered conventional.” P-hacking and hypothesis fishing are rewarded because they make for more remarkable, and therefore more publishable, results (Figure 2).
The legal system, by contrast, is obsessed with questions of proof and evidence. Lawyers have rigid structures to test the hypotheses underlying a legal battle. Lawyers are trained to study and argue both sides of a case, carefully finding holes in each. Evidence unrelated to a case can be thrown out by a judge, who is supposed to be an unbiased referee of competing claims. Science is inconsistent, with ad hoc rules, in comparison.
The U.S. judicial system is not a paragon, but pivotal breakthroughs in understanding usually come at points of convergence between two seemingly unrelated subjects. Appreciating the epistemological differences between science and law may give researchers and policy experts a new set of tools for thinking through science’s problems.
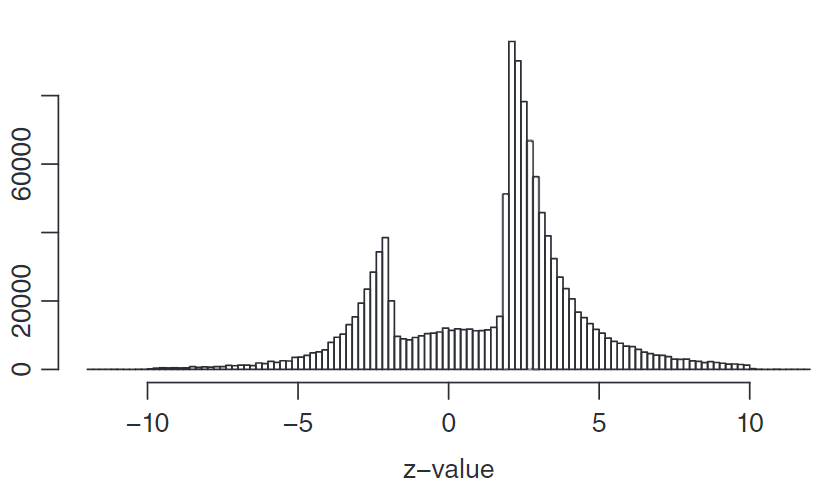
Consequences
The legal system is built on consequences. The wrong decision can ruin lives. If a judge is not impartial, or a defense attorney is incompetent, an innocent person goes to prison. A ‘remarkable’ result based on biased truth-finding or planted evidence is a wrongful conviction. The stakes are high.
In science, consequences for wrongdoing seem rare. I know this from experience because my first writing gig was at Retraction Watch.
While there, I reported on many scientists who did ethically dubious things — they published papers with manipulated images, served as a guest reviewer at a journal and only accepted papers that cited their work, or touted (ineffective) COVID drugs while failing to disclose familial or financial ties to the drug’s manufacturer. None of these people, to my knowledge, have been punished.
Even when editors are presented with clear evidence of data manipulation, they can take years to issue a simple expression of concern. In some cases, blatant data manipulation is punished with a slap on the wrist, or authors are asked to publish a correction to their (undermined) study. The current paradigm for dealing with misconduct is to preserve the integrity of the scientific literature, rather than punish individuals.
A veteran UCLA researcher, Janina Jiang, faked data in 11 different grant applications, 3 of which were funded for $58.7 million, according to reporting by Retraction Watch. The punishment? “Three years of supervision for any federally funded work.”
Jiang still appears to be employed at a UCLA affiliate hospital.
Evidence
The legal system has a nuanced view of evidence. There are special rules that dictate what and how evidence is presented, or which evidence is sufficient to establish proof in a case. Evidence can be thrown out entirely if it prejudices the jury, or was obtained through unlawful means.
There is transparency in the legal system, and that is important. It's not enough that the right answer is found; the process by which it is found must also be correct. Usually, that means how evidence was collected must be transparent and well-documented — something that the NHLBI only came around to in 2000 (Figure 1).
Most of science, by contrast, still runs on the “honorable gentleman” system. Admissibility of evidence is basically, "You know it when you see it.” Some academics give the pharmaceutical industry a bad rap, but it’s clear that the latter’s standards for evidence are far higher. Much like the law has codified processes and rules for (legal) trials, large biotechnology companies have rigid, formal procedures for their own (clinical) trials.

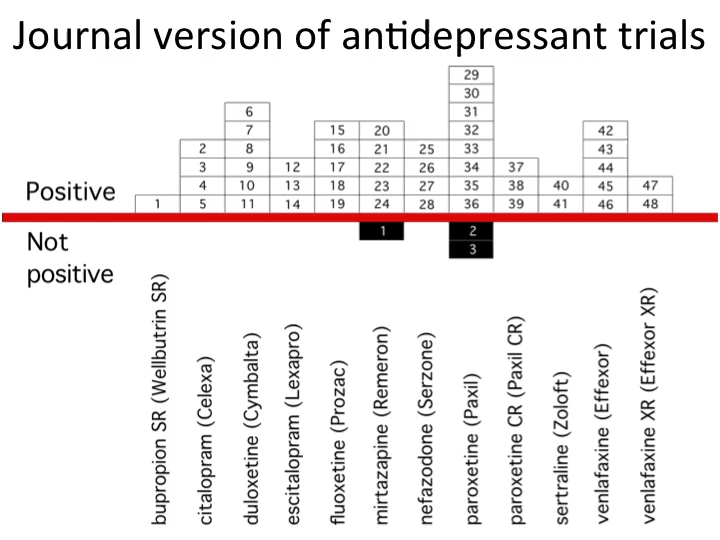
Biotechnology companies follow rigorous rules because they know their data must be scientifically sound — at some point, the FDA will snoop around. Data must hold up, or the company can’t reap financial rewards.
Academic scientists, by contrast, garner money, prestige, and tenure through short-term impacts and papers. Reviewers rarely have time to fact-check a paper’s data analysis or statistical methods, and most grants are awarded to scientists for short-term, “sure-to-succeed” research proposals.
Many scientists are familiar with the 2005 Ioannidis paper, and its claims that the majority of published “research findings are false.” But the consequences of such falsehoods are less appreciated and lead to billions of dollars in waste each year. A decade ago, the drug company Bayer halted “nearly two-thirds of its target-validation projects because in-house experimental findings [failed] to match up with published literature claims,” according to a news piece in Nature Reviews Drug Discovery.
Besides fraught standards for collecting evidence, academics are also less likely to report evidence compared with drug companies (at least, when it comes to clinical trials). The Mayo Clinic was compliant with FDA reporting rules for just 21.3% of trials, according to 2020 data from Ben Goldacre. The National Cancer Institute (which is part of the NIH) was compliant for 30.4% of trials. Pfizer? 92.9%. Novartis? 100%.
The U.S. Food and Drug Administration can impose hefty fines on non-compliant sponsors who do not upload results from a clinical trial. But they don’t, and they continue to award grants to researchers who break the rules. The standards between academia and industry are vastly different.
Adversaries
The American, English and Canadian legal systems are inherently adversarial. Lawyers argue for their clients, and the truth is thought to emerge from this struggle dialectically. Disputes are settled by ‘impartial’ judges.
Good lawyers, famously, know how to argue both sides of a case. They are trained in this art form early in their career; usually in the first year of law school. The best lawyers craft steel-man arguments to sharpen their case — they build up the best form of an opponent’s argument to find holes in its logic. A trial lawyer’s sense of truth, then, is often provisional.
An ideal scientist is her own worst critic, and is receptive to oppositional arguments. But debates seem quite rare at the institutional level. Domineering voices are all too common in NIH study sections, and “uniform” ideas often emerge victorious. Such siloed thinking can stifle progress.
Consider the Alzheimer’s cabal, a cadre of scientists who believe that beta-amyloid accumulation in the brain causes Alzheimer’s. To support their argument, they shut down competitors, rejected grant applications, and doled out money to friends from within NIH study sections.
In 2006, a Nature paper claimed that Aβ*56 is associated with cognitive decline. But recently, a six-month investigation by Science found “strong support” for image tampering by its lead author, Sylvain Lesné. The consequences? Lots of investigations, scant decisions, and many “no comments”. Bureaucracy as usual.
And the ongoing Alzheimer’s story is one fish in a roiling sea. In biomedicine, an estimated “3.8% of published papers contained problematic figures, with at least half exhibiting features suggestive of deliberate manipulation,” according to a 2016 analysis of more than 20,000 studies from 40 journals.
It’s unfortunate that, in study sections, many researchers are overwhelmed and simply don’t have time to consider dozens of proposals and debate their merits. Early-career faculty, perhaps cognizant of career suicide, are often hesitant to oppose decisions by senior faculty. And moving NIH peer reviews to Zoom, due to COVID, caused about 30 percent of scientists to contribute even less to discussions.
Margins
“Everything runs downstream from culture.”
– Tyler Cowen
Progress is made by small improvements to culture. Some scientists p-hack, but at least now we talk about it. Publication bias is still a major problem, but at least it’s recognized by editorial boards. Data sharing is still a problem, but at least journals are shifting policies. It seems we are on the right track.
But problems in science are hard to solve because they are cultural, and culture is dominated by incumbents who benefit from the status quo.
In 1930, legal scholar Karl Llewellyn published a book called, “The Bramble Bush.” In it, he argues that the officials in charge — sheriffs, clerks, judges — do more than just settle disputes; they dictate the law itself. Bloomberg columnist Matt Levine’s thoughts on Llewellyn is prescient for science and its problems, too (emphasis my own):
“...I went to law school. And I took the first-year course in Constitutional Law, and I learned about the fundamental principles that rule the United States. And I learned -- or at least was given the general impression -- that…the Constitution has served as a wise guide and constraint on the power of our rulers, and the foundation of our system of government. But in the back of my mind I thought about Llewellyn. I thought about the fact that those principles can't automatically enact themselves, that they only work if the human actors in the system choose to follow them and to demand that others follow them. They persist because the people constrained by them believe themselves to be constrained by them…Their magic is fragile, and can disappear if people who don't believe in it gain power.
Improving science takes time, and will demand that individuals who believe in the truth, and want to get at the truth, acquire power. A new generation of ideas must strangle the old.
Pre-registered hypotheses should be a requirement for just about every experiment. Scientific misconduct should be punished. Domineering voices should be removed from NIH study sections.
In a hundred years, I suspect our grandchildren will look back at our modern, scientific landscape and laugh at academia’s cavalier attitude towards rigorous statistical training, p-hacking, and hypothesis switching. Many studies are irreproducible and poorly designed. The whole system seems Victorian in its regard to prestige and social status, much like the legal system 150 years ago.
But if scientists behaved a bit more like lawyers, at least on the margins, perhaps we’d build a better future for those grandkids all the same.
Thanks to Kian Faizi, Benjamin Reinhardt, Adam Strandberg, Brian Finrow, Alexey Guzey and Sasha Targ for reading this.
Cite this essay:
McCarty, N. "The Laws of Science" newscience.org. 2022 August. https://doi.org/10.56416/721mpl
I think this statement, "Pre-registered hypotheses should be a requirement for just about every experiment", seems incorrect because it implies that all experiments are hypothesis driven. While that may be the case in some fields, how can that be the case for something like an experiment which characterizes the binding curve of a protein.